In an increasingly regulated economy, finding and capitalizing on the common denominator between news laws, directives, standards, or best practices is crucial. Using a megamodel is the best way to build, maintain and evolve an efficient cross-regulation platform, avoid the multiplication of data silos and prevent systemic risks from occurring, according to Fabien Henriet, Global CTO at eProseed RTC.
In an increasingly regulated economy, finding and capitalizing on the common denominator between news laws, directives, standards, or best practices is crucial. Using a megamodel is the best way to build, maintain and evolve an efficient cross-regulation platform, avoid the multiplication of data silos and prevent systemic risks from occurring, according to Fabien Henriet, Global CTO at eProseed RTC.
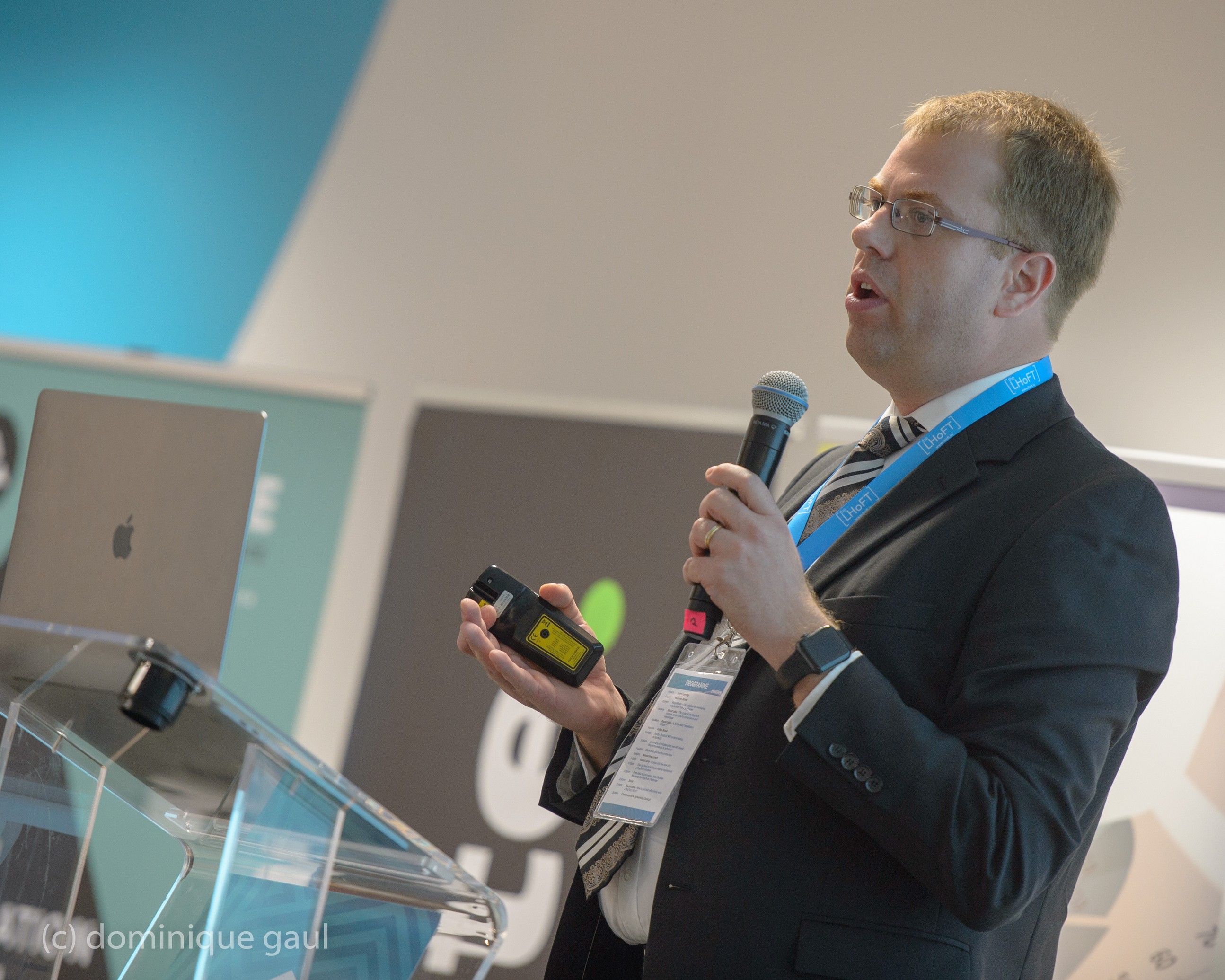
Regulation, a Growth Industry
“We are rapidly moving towards a regulated world. Since the 2008 global financial crisis, an increasing number of laws, rules, regulations and incentives are being issued every year by different supervisory authorities at the national, regional and international levels.
This increased propensity aims at closing loopholes in the financial regulatory framework”, Fabien Henriet told the audience attending the second RegTech Summit Luxembourg, hold on October 11th at the Luxembourg House of Financial Technology.
This trend is not specific to the financial world: all industries, all sectors of activity are concerned. “With the introduction of new regulations such as GDPR, PSD2 or NIS, we cannot deny that we live in a regulatory world, to the point that regulation now appears to be a growth industry”, said Fabien Henriet.
Indeed, according to the 2018 edition of Thomson Reuters’ Cost of Compliance 2018 survey, compliance officers from the financial sector are experiencing regulatory fatigue and overload in the face of ever-changing and growing regulations. The report also shows that 69% of the firms surveyed – including banks, brokers, asset managers and insurers – are expecting regulators to propose even more rules in the coming year. And Thomson Reuters draw the same conclusions for the healthcare sector.
The Booming of Dedicated Data Repository Systems
The increase of regulations has highlighted and reinforced the issues faced by all organizations, regulated companies as well as regulators. Those challenges – fragmented IT tools, data heterogeneity and the burden of their collection, not to mention budget constraints and limited resources – have led to a need for collecting all data into dedicated data repository systems intended to provide a reliable and unique source of data, establish data lineage, data governance and data quality as requested by regulations such as BCBS 239, and ultimately reduce regulatory overload.
“Such data repository systems rest on two main pillars”, Fabien Henriet explained. “The first one is process automation, that is to say limiting as far as possible human interventions. The other one is data analysis. Some of these solutions are suitable for regulated entities as well as regulators but, actually, they are rarely shared between the two parties. And even if some platforms feature truly advanced complexity in terms of analytics, AI or predictive modelling, they are specific to one single regulation.”
However, one organization may be subject to several regulations – which is hard to manage with different data repositories – while some regulations may apply to all sectors, such as data protection and information security laws. As a result, using dedicated data repositories leads to data duplication, consistency issues, and difficulties in establishing an overall view of risk management and governance.
“This leads to the creation of monolithic architectures that turn out to be unmaintainable. This is due to the fact that trying to encompass more regulations, more laws, more best practices, means extending the model to the point where it would become a supermodel capable of comprehensively analyzing and understanding literally everything. And this simply does not work”, stated Fabien Henriet.
Next Step: Megamodel
“To overlay regulations into a 360° view, what we need first and foremost, rather than technological platforms, is a comprehensive theory mixing up into one single picture analytical, simulation, data driven, and ontological models”, he added. “This comprehensive scheme, called megamodel, is aiming to find the intersection between different models – between elements of regulations in our particular case”.
According to the definition given by the Global CTO of eProseedRTC, a megamodel is a collection of metamodels, models, data models, designed for data acquisition, composition, integration, management, querying, and mining. Therefore, a megamodel-based platform could offer a 360° view of the regulatory landscape. Such a solution would be capable of mastering the co-evolution of data and models – for instance by integrating jurisprudential decisions – and of supporting the creation of what-if analysis, predictive analytics and scenario explorations, whatever the data or sector under regulation.
Anticipating Systemic Risk
Among the benefits associated with megamodelling, Fabien Henriet has pointed out the possibility to estimate how the different risks are interconnected inside a given organization, evaluate how different organizations are interconnected and assess the likelihood of propagation of a risk from one organization or sector to another.
The risk of a major system failure causing a domino effect is called systemic risk. The main characteristic of such an event is that a risk is likely to spread from unhealthy institutions to relatively healthier institutions through a transmission mechanism. This term is used mainly in the financial sector, but it can also apply to any industry in our interconnected world.
“Megamodel is the model of models capable of modelling complex relationships and helping to evaluate the potentiality and impact a risk propagation, automatically and across sectors”, Fabien Henriet concluded.